The world of machine learning is continually evolving and advancing, and with it comes new ways to develop and deploy machine learning models. Machine Learning as a Service (MLaaS) is a type of cloud computing service that enables businesses to build and manage machine learning models without requiring in-house expertise or resources.
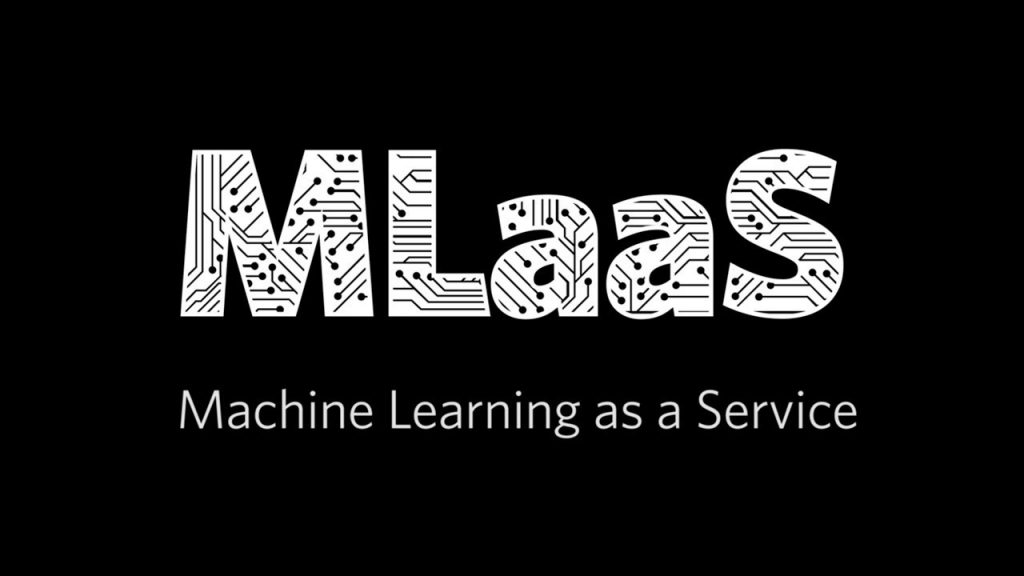
MLaaS makes it possible for businesses to deploy and experiment with machine learning models quickly and efficiently with a wide range of services and tools. Automated data labelling, model training and deployment, and model experimentation are just some of the features included in many MLaaS offerings. By removing the need for specialized in-house knowledge and resources, MLaaS makes it easier for organizations to benefit from the predictive insights that machine learning models can provide.
But what does MLaaS mean for the future of machine learning? For one, it’s likely that more businesses will start adopting MLaaS, as it simplifies the process of creating and deploying machine learning models. In addition, MLaaS can help organisations cut costs, as they no longer need to invest in expensive in-house expertise or resources.
It’s also possible that MLaaS will lead to more widespread use of machine learning. By making it simpler and more cost-effective to build and deploy machine learning models, MLaaS could open the doors to more organizations using machine learning, giving them access to the predictive insights that machine learning models can provide.
Finally, MLaaS could lead to more advanced machine learning models. As machine learning models become easier to experiment with and deploy, organizations can start exploring more complex models and using them to gain even greater insights into their business operations and customers.
Top MLaaS Platform
Some of the top MLaaS providers in the market are:
Microsoft Azure Machine Learning Studio is a cloud-based data science platform that allows users to build and deploy machine learning models using a visual programming environment. It provides users with a variety of tools and services to make developing machine learning models easier and more efficient. These features include cloud-based data storage, automated model selection and optimization, and a variety of visual tools to facilitate the development process. Additionally, it includes services such as automatic data preparation and model deployment, allowing users to quickly deploy their models and get predictive insights from their data.
Amazon Web Services (AWS) Machine Learning is a suite of cloud-based services that make it easier for organizations to develop, deploy, and manage machine learning models. It includes features such as automated data labelling, model training, and deployment, allowing organizations to easily deploy their models and get predictive insights from their data. It also includes services such as managed machine learning workflows and model experimentation, allowing organizations to quickly experiment with different models and find the one that best suits their needs. Additionally, it provides a variety of tools and services to help organizations manage the cost of their machine learning projects.
IBM Watson Machine Learning is a cloud-based AI platform that enables organizations to create, deploy, and manage machine learning models. It provides users with a variety of tools and services to make developing machine learning models easier and more efficient. These features include automated model selection and optimization, cloud-based data storage, and a variety of visual tools to facilitate the development process. Additionally, it includes services such as automatic model deployment and predictive analytics, allowing organizations to quickly deploy their models and get insights from their data.
Google Cloud Machine Learning Engine is a hosted platform for running machine learning training and predictions at scale. The service treats these two processes (training and predictions) independently, and it is possible to use Google Cloud ML Engine just to train a complex model by leveraging the GPU and TPU infrastructure. The outcome from this step, a fully-trained machine learning model that can be hosted in other environments, including on-prem infrastructure and public cloud. Cloud ML Engine automates all resource provisioning and monitoring for running the jobs, and can also manage the lifecycle of deployed models and their versions. Apart from training and hosting, Cloud ML Engine can also perform hyperparameter tuning that influences the accuracy of predictions. Additionally, it provides RESTful services for managing jobs, models, and versions, and for making predictions with hosted models on Google Cloud. It also supports a wide range of ML frameworks and processors, making it an ideal platform for ML applications.
BigML is an online platform for creating and managing predictive models and machine learning workflows. It provides users with all the tools necessary to build, evaluate, and deploy predictive models quickly and easily. BigML’s platform consists of a drag-and-drop interface that allows users to create models and workflows in minutes. It also provides an API that allows developers to integrate their models into applications. The platform also supports a wide variety of programming languages and data sources, making it easy for developers to get started quickly. BigML also includes a number of features such as automated model optimization, user-defined goals, and pre-built models for common tasks such as sentiment analysis. BigML is a great platform for quickly creating and managing predictive models and workflows.
H2O.ai is an advanced AI Cloud Platform designed to simplify and accelerate making, operating and innovating with AI in any environment. It is the company behind open-source Machine Learning (ML) products like H2O, aimed to make ML easier for all. H2O.ai is the leading AI cloud company, on a mission to democratize AI for everyone. Customers use the H2O AI Cloud platform to rapidly make, operate and innovate with AI in any environment. The platform provides a range of services and tools to help users create and manage AI models and applications. Additionally, the platform offers a demo service to help customers get started using the platform.
Beginner Tips
If you are a beginner to MLaaS, it is recommended to start with one of the more user-friendly platforms, such as Amazon Web Services (AWS) or Google Cloud Platform (GCP). Both platforms offer comprehensive ML services with a range of options. AWS, for example, offers SageMaker, which provides an easy-to-use development environment with automated model tuning and deployment capabilities. GCP, meanwhile, offers Cloud ML Engine, which is a hosted platform for running machine learning training and predictions at scale. Both platforms also provide a range of tutorials and resources to help users get started quickly. Additionally, both AWS and GCP offer a free-tier service that allows users to experiment with MLaaS without having to pay any upfront costs.
When experimenting with MLaaS, it is important to consider the costs associated with the platform, as these can add up quickly. Additionally, if you are just starting out, it is recommended to begin with simpler problems and gradually work your way up to more complex tasks.
Furthermore, it is important to familiarize yourself with the capabilities offered by a particular platform, as well as its limitations, before you start using it for any serious tasks. Finally, always be sure to read the documentation for the individual services, as this will help you gain a better understanding of how to use them.
Conclusion
The future of machine learning is ever-evolving, but one thing is for sure: MLaaS is playing an important role in the future of the field. By making it easier and more cost-effective to develop and deploy machine learning models, MLaaS is helping organizations unlock the potential of machine learning, gaining valuable predictive insights and improving their operations.